Russell S. Schwartz
Professor and Head, Computational Biology Department
Address:
654B Mellon Institute
Department of Biological Sciences
Carnegie Mellon University
4400 Fifth Avenue
Pittsburgh, PA 15213
Phone: 412-268-3871
Fax: 412-268-7129
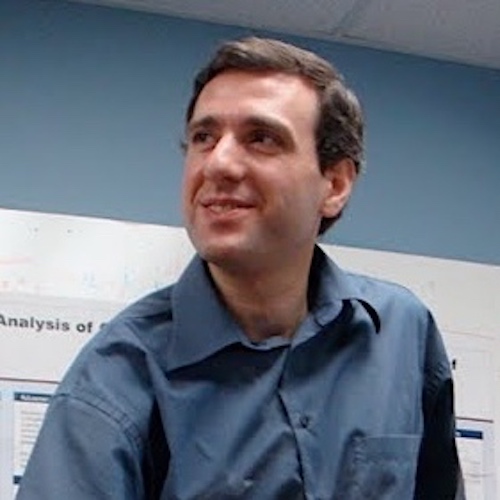
Education
Ph.D., Computer Science, Massachusetts Institute of Technology
Postdoctoral Appointment, Massachusetts Institute of Technology
Research
My group works broadly in algorithms, machine learning, and numerical methods for understanding complex systems in biology. We have worked on many topics in computational genetics, genomics, and biophysics. In the genetics direction, this has included contributions to genetic variation analysis, phylogenetics, and population genetics. In biophysics, our work has been focused on stochastic simulation, particularly of macromolecular assembly processes, and related methods in model inference. The majority of our recent work is focused on somatic evolution and its connections to cancer. This includes work on algorithms for reconstructing cellular lineages in cancers (“tumor phylogenetics”) and machine learning for predicting progression outcomes.
Particular recent areas of focus have been multiomic data integration, productively using single-cell and spatial genomics platforms, and blood based tumor genomics (“liquid biopsy”).
I am also active in computational biology education. This work is especially focused on how to set standards and define core competencies and best practice for computational education in the life sciences. Areas interest include defining the standards for education of computational biology specialists as well as identifying needs for computational training among experimental biologists, health professionals, and other members of the life sciences community.
Publications
The following are selected recent publications:
Sc-TUSV-ext: Single-cell clonal lineage inference from single nucleotide variants (SNV), copy number alterations (CNA) and structural variants (SV)
N.A. Bristy, X. Fu, R. Schwartz. Journal of Computational Biology, accepted for publication, 2025
Optimizing design of genomic studies for clonal evolution analysis
A. Srivatsa and R. Schwartz. Bioinformatics Advances, 4(1):vbae193, 2024
The ISCB competency framework v. 3: a revised and extended standard for bioinformatics education and training
C. Brooksbank, M.D. Brazas, N. Mulder, R. Schwartz, V. Ras, S.L. Morgan, M. Lloret-Llinares, P. Carvajal-López, L. Larcombe, A. Ghouila, T. Hancocks, V. Satagopam, J. De Las Rivas, G. Mazandu, B. Gaeta. Bioinformatics Advances, 4(1):vba126, 2024
Modeling the effect of spatial structure on solid tumor evolution and ctDNA composition
T. Rachman, D. Bartlett, W. LaFramboise, P. Wagner, R. Schwartz, O. Carja. Cancers, 16(5), (Special Issue on Circulating Cancer Biomarkers: Progress, Challenges and Opportunities), 2024
Determining Optimal Placement of Copy Number Aberration Impacted Single Nucleotide Variants in a Tumor Progression History
C.-H. Wu, S. Joshi, W. Robinson, P. F. Robbins, R. Schwartz, S. C. Sahinalp and S. Malikic. Proc. Research in Computational Molecular Biology (RECOMB), 2024
Grand challenges in bioinformatics education and training
E.B. Işık, M.D. Brazas, R. Schwartz, B. Gaeta, P. M. Palagi, C.W. G. van Gelder, P. Suravajhala, H. Singh, S.L. Morgan, H. Zahroh, M. Ling, V.P. Satagopam, A. McGrath, K. Nakai, N. Mulder, C. SchönbachY. Zheng, J. De Las Rivas, A. M. Khan. Nature Biotechnology, 41:1171-1174, 2023
A clonal evolution simulator for planning somatic evolution studies
A. Srivatsa, H. Lei, and R. Schwartz. Journal of Computational Biology, 30(8):831-847, 2023
Ten Simple Rules for Writing a PLOS Computational Biology Quick Tips Article
P. Palagi, R. Schwartz, F. Ouellette, S. Markel. PLoS Computational Biology, 19 (12), e1011689, 2023
Interpretable deep learning for chromatin-informed inference of transcriptional programs driven by somatic alterations across cancers
Y. Tao, X. Ma, D. Palmer, R. Schwartz, X. Lu, H. Osmanbeyoglu. Nucleic Acids Research, 50(19):10869-10881, 2022
Reconstructing tumor clonal lineage trees incorporating single nucleotide variants, copy number alterations, and structural variations
X. Fu, H. Lei, Y. Tao, and R. Schwartz. Bioinformatics (ISMB proceedings issue), 38 (Supplement_1), i125-i133, 2022
Semi-deconvolution of bulk and single-cell RNA-seq data with application to metastatic progression in breast cancer
H. Lei, X. Guo, Y. Tao, K. Ding, X. Fu, S. Oesterreich, A.V. Lee, and R. Schwartz. Bioinformatics (ISMB proceedings issue), 38 (Supplement_1), i386-i394, 2022
De novo prediction of cell-drug sensitivities using deep learning-based graph regularized matrix factorization
S. Ren, Y. Tao, K. Yu, Y. Xue, R. Schwartz, and X. Lu. Proc. Pacific Symposium on Biocomputing, pp. 278-289, 2022
ConTreeDP: A consensus method of tumor trees based on maximum directed partition support problem
X. Fu and R. Schwartz. Proc. 2021 IEEE International Conference on Bioinformatics and Biomedicine (IEEE BIBM 2021), pp. 125-130, 2021
Joint clustering of single cell sequencing and fluorescence in situ hybridization data for reconstructing clonal heterogeneity in cancers
X. Fu, H. Lei, Y. Tao, K. Heselmeyer-Haddad, I. Torres, M. Dean, T. Ried, R. Schwartz. Journal of Computational Biology, 28 (11), 1035-1051, 2021
Tumor heterogeneity assessed by sequencing and fluorescence in situ hybridization (FISH) data
H. Lei, E. M. Gertz, A. A. Schaeffer, X. Fu, Y. Tao, K. Heselmeyer-Haddad, I. Torres, G. Li, L. Xu, Y. Hu, K. Wu, X. Shi, M. Dean, T. Ried, R. Schwartz. Bioinformatics, 37 (24), 4704-4711, 2021
3D collagen vascular tumor-on-a-chip mimetics for dynamic combinatorial drug screening
J. Wan, Y. Yin, J. Skoko, R. Schwartz, M. Zhang, P.R. LeDuc, CA Neumann. Molecular Cancer Therapeutics, 20(6) 1210-1219, 2021
Assessing the contribution of tumor mutational phenotypes to cancer progression risk
Y. Tao, A. Rajaraman, X. Cui, Z. Cui, J. Eaton, H. Kim, J. Ma. and R. Schwartz. PLoS Computational Biology, 17(3), e100877, 2021